3.13 Long-Memory Stochastic Volatility Model
More recently, the SV model is further extended to allow for long memory in volatility, using the idea of fractional difference. As stated in Chapter 2, a time series is a long-memory process if its autocorrelation function decays at a hyperbolic, instead of an exponential, rate as the lag increases. The extension to long-memory models in volatility study is motivated by the fact that the autocorrelation function of the squared or absolute-valued series of an asset return often decays slowly, even though the return series has no serial correlation; see Ding, Granger, and Engle (1993). Figure 3.10 shows the sample ACF of the daily absolute returns for IBM stock and the S&P 500 index from July 3, 1962, to December 31, 2003. These sample ACFs are positive with moderate magnitude but decay slowly.
Figure 3.10 Sample ACF of daily absolute log returns for (a) S&P 500 index and (b) IBM stock for period from July 3, 1962, to December 31, 2003. Two horizontal lines denote asymptotic 5% limits.
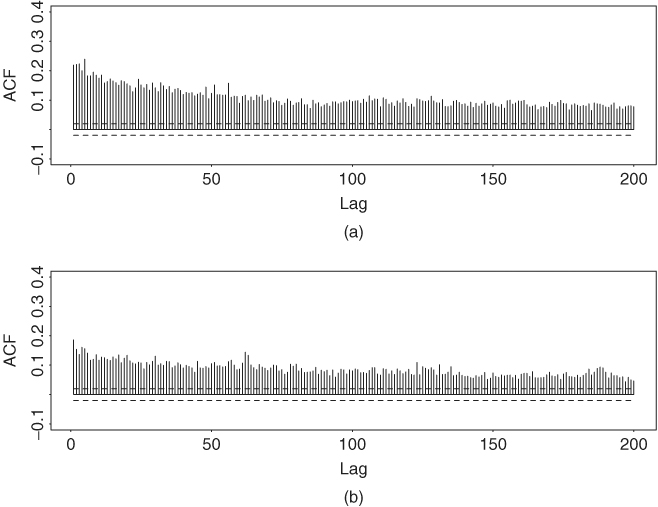
A simple long-memory stochastic volatility (LMSV) model can be written as
where σ > 0, the ϵt are iid N(0, 1), the ηt are iid and independent of ...
Get Analysis of Financial Time Series, Third Edition now with the O’Reilly learning platform.
O’Reilly members experience books, live events, courses curated by job role, and more from O’Reilly and nearly 200 top publishers.